J Biomed 2019; 4:44-54. doi:10.7150/jbm.35760 This volume Cite
Research Paper
Revealing Stroke Analysis Markers for Studying Heterogeneous Disease State of Stroke
1. University of Kentucky, Lexington, Kentucky 42101. USA
2. Manipal University, Manipal, Karnataka 576104, India
3. Kentucky State University, Frankfort, Kentucky 40601. USA
Received 2019-4-15; Accepted 2019-10-11; Published 2019-11-9
Abstract
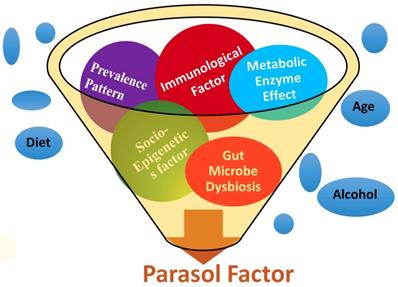
Early diagnosis of Stroke is challenging due to a lack of dependable diagnostic tests. Currently, the mainstay in early detection is by monitoring health an individual through traditional risk factors such as hypertension. Seeking new determinants, relevant to millennial life style, is therefore warranted. This study reveals new Stroke Analysis Markers (SAM) including Phosphodiesterase-4D involved in cardioembolic stroke. In addition to traditional factors, new millennial risk factors such as molecular and cellular determinants were studied based on 68 years of stroke research data from 1951 to 2019. The rs152312 SNP from stroke patients of deCODE was queried in eNSEMBL, BLAST and other databases to study PDE4D isoforms. In addition, the role of infection, immune cells, inflammation, gut microbial dysbiosis, the prevalence pattern of stroke in geographically different populations were analyzed. This study identified five new millennial risk factors as potentially helpful Stroke Analysis Markers for stroke by conjoining them as a single set of five parasol factors. They include genomic, microbiologic, immunologic, socio-epigenetic factors including two contig and alternative splicing markers along with their prevalence patterns among various populations. Taking appropriate preventive management by monitoring these new risk factors in high-risk individuals during annual checkup could help physicians to make an informed decision. Though significant challenges remain to be solved further large-scale studies on parasol factors will certainly unlock the secrets of early prediction of stroke.
Keywords: Stroke, Stroke Analysis Markers, Phosphodiesterase-4D
Introduction
Stroke is the second highest cause of mortality worldwide [1] and the fourth leading cause of lost productivity by humans globally [2]. In the US, every two minutes three people experience a stroke and every four minutes one dies of the stroke [3]. It is estimated that by 2030, about 23 million people will get a stroke for the first time [4]. Stroke is a potentially irreversible and presents with features of paralysis. Clinical observation with brain-imaging is useful for stroke diagnosis, but fails to be a useful predicting tool with regards to morbidity and mortality. In the past, several risk factors were attempted and tested as a measurable underlying process-based molecular marker to predict the possibility of a potential stroke event. Though predicting a stroke event in advance before it happen was unsuccessful, these factors rather helped physicians to predict recovery and/or treatment responses. The need for the development of a reliable tool to both improve prompt diagnosis and to monitor progression and prognosis is thus an urgent need.
Years of research in stroke revealed several risk factors including hypertension in 1951 [5] to MicroRNA-15a/16-1 cluster in plasma in 2017 [6]. After studying hundreds of stroke data published over the past 68 years from 1951 to 2019, it is clear that the majority of those studies describe a few stand-alone risk factors for stroke. Some examples include hypertension [7], atherosclerosis [8], diabetes [9], large artery occlusion [10], hypercholesterolemia [11], administration of alteplase [12], Tissue plasminogen activator [13], cigarette smoking [14], influence of Statin drug [15], air pollution [16, 17], and socio-economic issue like poverty [18].
Several epidemiological studies like Framingham Heart [5], Task Force for stroke prevention [19], Stroke Buckle [20], CHS [21], Stroke Belt [22], Jackson Heart [23], EUROSTROKE [11], ARIC [8], REGARDS [24], NHANES [25], INTERSTROKE [26], Qstroke score [27], GBD [16], ACC/AHA [28], FSRS [29], ENCHANTED [12, 30], STROKOG [31], Stroke care system in Asia [32], Brazilian guidelines [33], and many more revealed the correlation between community, life-style, socio-economic and traditional risk factors. Some studies relied on single to multiple survey reports, while others reported data with or without periodic follow-ups. It is thus imperative to recalibrate traditional risk factors with new factors from millennial research fields such as genomics, proteomics, metabolomics, epigenetics, etc., to identify new sets of Stroke Analysis Markers (SAM). Evidently it is expected that these SAMs will help researchers to develop custom-made novel prognostic tools for identifying heterogeneous disease state of Stroke during a potential strokegenesis.
The term 'Stroke' was first scientifically recognized in 1965 in place of 'apoplexy' [34]. Once it was removed from the group of heart diseases and classified as an independent health disorder, stroke research gained momentum since then by focusing on the traditional risk factors. However a fundamental shift was made as early as 2003 by the deCODE study, which found the enzyme Phosphodiesterase 4D (PDE4D) as a sole risk factor for stroke in Icelanders [35]. Since then, several new millennial risk factors were overwhelmingly put-fourth, as meaningful and measurable Stroke Analysis Marker (SAM) to understand this heterogeneous disease. The present study reviewed these metadata, to find most reliable millennial risk factors for stroke prognostic purposes.
Although the objective of the current study is to collect data on millennial risk factors beyond traditional ones, the ultimate aim is to identify a set of reliable SAMs for a potential heterogeneous state of stroke well before the traditional clinical threshold enabling physicians and patients to deal and to be ahead of occurrence of a stroke event. As reported earlier, “in practical terms, biomarkers should improve our ability to predict long-term outcomes after stroke across multiple domains” [36]. Keeping this in mind, the current study was designed with following hypothesis; (i) collecting research data and results from the last 66 years of stroke studies since the first stroke study in 1951 could benefit my millennial factor search in stroke research; (ii) finding the PDE4D gene and its isomers in chromosomes could augment the predictability of stroke occurrence based on its expression; and (iii) revealing reliable causative factor(s) by performing a meta-analysis with multiple comparisons of datasets could lead to a prognostic tool for predicting potential PDE4D-associated cardioembolic stroke.
Methods
Bioinformatics data acquisition and Challenges
This research was carried out at Bioinformatics and Computational Biosciences of NIAID-NIH, USA. This study was conducted based on the published data from traditional and millennial risk factors and stroke-related research data obtained from 66 years of stroke studies. The published research outcomes were directly gathered from Bethesda main campus library of NIH and through on-line NIH multi-eLibrary portals including proverbial websites as given in the Table 1. This unique opportunity to work at NIH helped authors to use, and reuse with published data and mingle with stroke researchers in order to explore the significance of the stroke biomarkers and its outcomes. Analyzing several noteworthy research publications for seeking new set of molecular and cellular determinants, especially relevant to millennial life style was a key challenge in this study. Followed by the literature-search, the conventional bioinformatics analysis and processes including the BLAST search of the probe rs152312 were carried out. With data harmonization, several Stroke Analysis Markers were identified and categorized as a few sets of parasol factors.
Data sets and attainment of parasol factors
Stroke etiology thus far has proven to be based upon a collection of traditional risk factors such as heart disease, diet, age, race, gender, medication, smoking, diabetes, stress, etc. Starting from the first stroke research in 1951, several types of literatures on stoke were initially searched in PubMed [37], and cross-referenced with several other web portals (Table 1). After analyzing more than six decades of stroke research data, the results were categorized into five groups such as (i) metabolic, (ii) immunologic, (iii) microbiologic, (iv) socioepigenetic, and (v) population-demographic risk factors. In addition, the prevalence patterns of stroke among the global population including the Stroke Belt and Stroke Buckle regions of US were assembled to develop a unique set of risk factors with five determinants under one umbrella as parasol factors (Figure 1).
Bioinformatics websites used and referred in this study
Millennial risk factors search
PDE4D belongs to the enzyme superfamily, Phosphodiesterase. It was selected as a new millennial factor because of (i) its association with stroke as identified by the deCODE project [35], (ii) its regulatory role by controlling another very significant pathological protein, cyclic adenosine mono phosphate or cAMP [38], and (iii) its multiple genetic variants which predict potential risk of a disease as a biomarker [39]. A unique Single Nucleotide Polymorphism genomic probe, 'rs152312 SNP-41' of PDE4D obtained from deCODE, was used in this study, because of its allele frequencies that were constructed from the genetics of stroke patients in deCODE study [40]. This probe was queried at '1000-genome project' browser [41], and in the ENSEMBL site [42], followed by OMIM database [43]. The socio-epigenetic effects of PDE4D and their prevalence patterns among populations were also studied. In addition, the role of microbiological and immunological factors in association with stroke was searched, since the immune system plays a very significant role in stroke development [44]. The effect of set of three immunological factors such as Infection, Immune cells and Inflammation, and a microbiological factor such as gut-microbiome were added as the fourth and fifth novel factors in association with strokegenesis (Figure 1). All websites referred in this study was tabulated (Table 1).
A pictorial expression of parasol factors involved in stroke: The millennial risk factors of this study were categorized into (i) metabolic effect including PDE enzyme factor, (ii) role of infection, immune cell and inflammation in stroke, (iii) microbiological factors such as the role of gut microbiome, (iv) socio-epigenetic factors and (v) population demography and prevalence pattern-based factors. In addition, though not included under parasol factors, several traditional risk factors were also studied [5 to 18], under the prevalence patterns of stroke in the global perspective including the Stroke Belt and Stroke Buckle in the US were assembled.
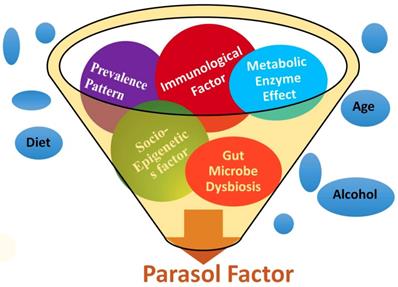
Results
Metabolic Factors
The BLAST search of the probe rs152312 returned with the PDE4D gene of stroke patients and correlated as a biomarker in chromosome 5, which is implicated in stroke. Within the PDP4D genome domain, a conserved sequence containing four short nucleotides, GAAA, was identified as a contig sequence for the first time in this study. Further phylogenetic analysis (as queried in the eNSEMBL browser) identified the contig sequence for the first time, as an evolutionarily conserved sequence found in several other primates (Figure 2). It proves a functional significance of the contig sequence. Further investigation on the Exome Sequence of PDE4D revealed 18 repeats of contig sequence (10 found on the exons and 8 found on the intron sequence of PDE4D). These 18 conserved contig sequences, uniquely present in PED4D, were identified for the first time as Alternative Splicing (AS) sequences in this study.
The bioinformatics study using single nucleotide polymorphisms (SNPs) probe: The probe rs152312 SNP-41 was selected, as it is distinctively pertained to stroke patients of Icelanders, from the published deCODE project [35, 40] and was used to BLAST at various bioinformatics websites (see details in table 1). The results revealed matching PDE4D, and correlated as one of the Stroke Analysis Marker (SAM) located in chromosome 5, which is implicated in stroke. Within the PDE4D genome domain, a conserved sequence containing four short nucleotides, GAAA, was identified as a contig sequence. Further phylogenetic analysis as queried in the eNSEMBL browser revealed matching sequences, which indicated the distribution of this conserved contig sequence in primates.
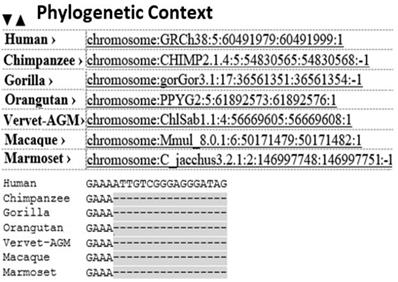
Effects of Infection, Immune, Inflammation and Gut-Microbe Symbiosis
The meta-analysis revealed the role of Infection, Immune and Inflammation in stroke (Figure 3). In an earlier study, higher levels of AS are noted in immune and nervous systems [45], with implications in inflammation [46]. It is crucial for the regulation of multi-domain proteins [47]. In the present study, presence of 18 AS sequences that were identified in PDE4D, confirming the association of PDE4D enzyme with Infection, Immune cell and Inflammation as risk determinants of strokegenesis.
Activation of brain by gut microbiome and the role of immunological factors: It was proved earlier that in an effective stroke event, gut microbes play role in influencing the event through activation of brain via signaling pathways through intestinal barrier [78, 97, 98]. It can be triggered by immunological factors such as an infection. Our analysis from 100s of published articles revealed the potential role of Infection, Immune cell and Inflammation in strokegenesis. The presence of 18 Alternative Splicing sequences of PDE4D that were identified in the current study, reveals the association of PDE4D enzyme with Infection, Immune cell and Inflammation as risk determinants of strokegenesis.
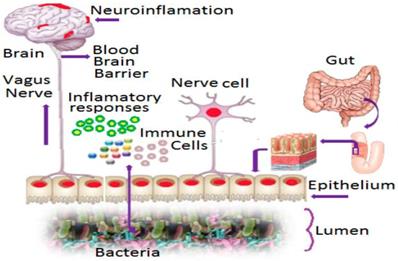
PDE4D gene and Epigenetic Readjustment
Alternative Splicing (AS) is associated with diversity of protein isoforms through micro-evolutionary processes that may play a role in disease in humans. Earlier studies report that new mutants of protein are more likely to be expressed at lower levels as their deleterious effects are more likely to be maintained if the original isoform is stable [48]. This could be one of the reasons that isoforms of PDE4D are found in stroke patients of the deCODE studies of Icelanders, but not in the studies of other regions. In our study, the presence of PDE4D across the species was revealed through the Sunburst visual tree data (Figure 4). The phylogenetic BLAST analysis identified the contig sequence of GAAA in primates, and extensive numbers of 18 AS markers were found in the PDE4D sequence. It was reported earlier that, splice variant expression is regulated in several ways, developmentally, spatially, and in response to external stimuli [49]. Therefore, the influence of risk factors, including AS variants of PDE4D, might have been inherited and expressed in Icelanders genetically and/or epigenetically, which explains the role of PDE4D in stroke prevalence in Icelanders and many other countries while not in others. For example, a recent study proved that a heat-shock gene is epigenetically expressed in some progenies but not in all, however, after several generations, the gene was normalized due to what is called “epigenetic readjustment” [50]. If epigenetic readjustment impacted PDE4D isoforms, that should be consistent with effects in other diseases or phenotypes in at least some generations of Icelanders, and not limited to stroke alone. Interestingly, the OMIM record shows that the PDE4D also triggers a rare disorder called Acrodysostosis-2 [51]. It means PDE4D could have epigenetic influences for stroke in Icelanders. When normalizing for several generations, it expressed different phenotypes of “stroke-like symptoms” likely due to isoforms of PDE4D.
Sunburst visual tree indicates the distribution of metabolic enzyme PDE: The presence of most influential Phosphodiesterase (PDE) across the species as arranged in a radial format with each taxonomic group in the circle. The PDE enzyme controls the level of cAMP and cGMP enzymes and they regulate many physiological functions [104] such as signal transduction, immune response, inflammation, neuronal activity, hypertension etc. Variant isoforms of this enzyme could be considered as the single most responsible metabolic factor in causing neurological disorders such as stroke in certain populations as revealed in this study.
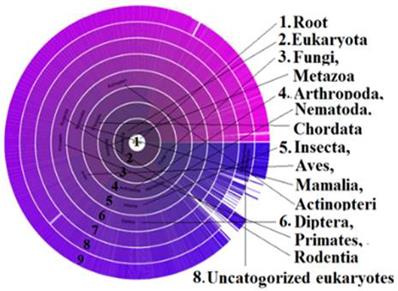
Prevalence Patterns in Population
The US stroke research studies revealed that out of 50 states, eight of them (Alabama, Arkansas, Georgia, Louisiana, Mississippi, North Carolina, South Carolina, and Tennessee) belong to the 'Stroke Belt' region (Figure 5), where the rate of stroke is higher compared to all other 42 states [22]. This pattern was noticed in l940 [52] and further recognized in 1960 [53]. Three states (North Carolina, South Carolina, and Georgia) showed yet another pattern called the 'Stroke Buckle,' where stroke mortality was twice as high as the other 47 states [20]. Meta-analysis confirmed that low socio-economic status was not a major factor other being more likely associated with hypertension [54] despite the higher use of anti-hypertensive medications in the Stroke Buckle [9]. Among all races and genders, Black men aged 40-59 had high rates of stroke episodes [7, 55]. Some studies such as the Jackson Heart Report [23] and the Framingham Stroke Risk Score from the REGARDS [24, 9] suggested that hypertension may be one of the highest risk factors. The main factor for high stroke mortality in the Stroke Buckle was a mystery 20 years ago [14], and still remains so today. Currently, states like Kentucky, West Virginia, and Indiana show higher mortality with stroke incidents but lie beyond the Stroke Belt, furthering the need for better quantification of risk factors and biomarkers.
Though strokegenesis occurs universally in all genders and races, in reality the distribution patterns of stroke are masked by factors like race, gender, and geographical distribution. In China, 7 million stroke cases were reported in one year, which is approximately a fourth of total stroke-related deaths in the world [56], though the pattern is not consistent across the globe, as in the case of the Russian Federation and Eastern Europe. Some African countries like Nigeria and Tanzania have shown an increased level of strokes [57]. When deCODE study showed the correlation of 87 single nucleotide polymorphisms (SNPs) of PDE4D with cardioembolic stroke [35] among Icelanders, a lack of correlation was observed in Mongol and Han populations in Mongolia [58]. Cohort studies in Germany [59] and in Korea [60] revealed genetic variants of ischemic stroke that differed within the population.
Stroke prevalence patterns in the United States: Several studies on stroke research in the United States revealed that out of 50 states, eight of them (Alabama, Arkansas, Georgia, Louisiana, Mississippi, North Carolina, South Carolina, and Tennessee) belong to the 'Stroke Belt,' where the rate of stroke is higher compared to all other 42 states. Among these eight states, three of them (North Carolina, South Carolina, and Georgia) showed yet another pattern called the 'Stroke Buckle,' where stroke mortality was twice as high as the other 47 states [20, 22]. Though it is not a geographically isolated island, why stroke has prevailed highly in this region was a mystery in 1951 study and remains a mystery today.
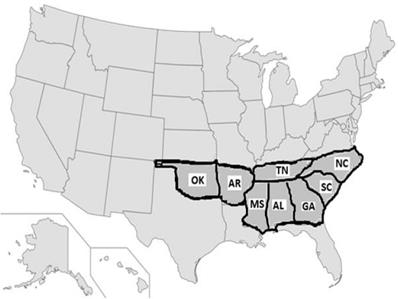
Discussion
Developing Parasol Factors for Stroke
Finding a single causative factor for stroke is not feasible as stroke is one of the most complex pathophysiological processes that affect humans. Though several biomarkers range from objective clinical tools like neuroimaging to wet-markers like blood and cerebrospinal fluid, no single diagnostic tool for stroke was discovered. However, the loss of millions of people due to stroke warrants urgent new strategies in stokes research and clinical planning. There is no doubt that the traditional risk factors play a major role in stroke; however, in reanalyzing the metadata of stroke studies from the last six decades, we found a few causative risk factors which play a role in strokegenesis. Among them, we identified five contiguous determinants as the most prominent millennial risk factors and conjoined them as a single set of parasol factors based on their interactive role in strokegenesis. Further studies will reveal the efficacy of this Stroke Analysis Markers (SAM) as prognostic tool to monitor cardioembolic stroke, well before a real-time stroke event occurs.
The metabolic PDE4D factor
Phosphodiesterase (PDE) is an enzyme that controls the level of cAMP and cGMP enzymes [61] and promotes vascular smooth muscle proliferation, vascular muscle migration, aggravates local inflammation, increases protein kinase C activation, and promotes Ca2+ inflow. These three enzymes regulate many physiological functions such as signal transduction, immune response, inflammation, neuronal activity, hypertension, memory, etc. [62]. Most importantly, an increased PDE4D gene expression can cause vital metabolic events such as atrial fibrillation and induction of cardioembolism [63]. The PDE4D enzyme has been proved as the single most responsible metabolic factor in causing stroke in certain populations [35]. Cardioembolic stroke is unique and different from other strokes. It occurs due to the obstruction of a brain blood vessel and subsequent damage to the brain tissue secondary to a clot traveling from the heart. PDE4D was the causative enzymatic factor for cardioembolic stroke in several hundred stroke patients in Iceland [64].
Socio-Epigenetic factors
Whether it is cardioembolic or another type, stroke is an irreversible disorder for a human and socio-economic disaster for a country. The estimated cost of dealing with Stroke in the US alone has reached $33 billion per year [3]. Understanding the genetic patterns of distribution among different socio-ethnic groups is vital for the future economy of a country. Though this study focused on PDE4D-associated cardioembolic stroke found in Icelanders, one cannot exclude the possibility of association with sequence variants of PDE4D in other regions of the world. Reanalyzing the socio-epigenetic factors of PDE4D in a population is therefore important. Alternative splicing, a type of need-based genetic engineering system hidden in DNA, generates diversity in different sets of functionally but structurally related proteins [65]. The fruit fly (Drosophila) has fewer genes than a roundworm, but can do more complex metabolic functions than primates because of the alternative splicing strategy during gene expression [66]. About 40 years ago, Gilbert predicted that alternative slicing acts like a “laboratory” to produce equal amounts of variants in any group of genes with similar patterns of new isoforms [67]. In other words, a single gene can generate a number of new isoforms of proteins as needed to carry out life functions as demanded. In the case of PDE, alternative splicing acts like a cell-factory and produces more than 60 variants of protein isoforms [68]. It was reported earlier that expression of AS-specific Extra-Cellular Matrix (ECM) genes has long been known to be an adaptive response of various cell types to mechanical stress [69]. The ECM is also involved in cell adhesion, cell-to-cell communication and differentiation by having a variety of molecules [70]. The enzyme PDE is located in the ECM, and its protein isoforms play a crucial role in disease and cellular stress through alternative splicing [71].
In 2001, the significance of genotypic and phenotypic relations of AS was reported however the consequences on the presence of extensive AS with identical protein isomer were not well studied [66]. This current study reveals 18 conserved AS sequences in PDE4D and relates the presence of extensive numbers of AS metabolic isoforms of PDE4D in the development of stroke and/or strokoid diseases. Some of these isoform domains of PDE4D are associated with a specific domain of the ECM, and the ECM genes were known to utilize AS for protein regulation [47]. In order for PDE4D to metabolically play a major role in stroke with cAMP, the AS must make multiple isomers for PDE4D. As reported earlier, proteins with numerous interactions may have multiple functions corresponding to high connectivity [72]. It is known that multiple isoforms of PDE4D are produced with other proteins such as AMP and GMP in the ECM. It is reasonable to state that if new transcription failed to perform its function at the site where it should be, replacement molecules are not likely to be identical, and by extension less likely to be functional [39]. Therefore, whatever AS generates via different mRNA [73], the resulting isoforms could encode functionally different protein disorders, as in the case of PDE4D. As a result of functional role AS, it can be used as one of the SAM probes and/or developed into a novel prognostic marker for PDE4D-associated stroke.
This study also found an interesting analysis that the extensive number of AS present in PDE4D has direct relationship with Icelanders. The SNP probe we analyzed in this study was 'rs152312 SNP-41' of PDE4D, obtained from deCODE [35, 40]. It revealed unique genetic variability outcome as reported earlier in the Icelander population [35, 40, 64], but not in some other populations such as German population [59], even though most of the Icelander's ancestors are of German descent. It could be possible that the 'founder effect' (isolated population) played a role in the Icelandic population, which restricted the gene pool, causing variable PDE4D isoforms expression [59]. Another interesting finding from our analysis is that a set of PDE4D inhibitors that is non-competitive with cAMP-binding sites was found in some stroke patients as per an earlier study [68]. This means specific binding sites on PDE4D are available for docking by proteins beyond the catalytic domain. This was not the case in a German population [59], but was found in Icelander's PDE4D as it was involved in cardioembolic stroke.
Prevalence Pattern factor
Several studies in the past clearly demonstrated that the stroke happens due to combinatorial risk factors. No single factor or combination of single resilient reasons for underlying pathogenesis of stroke was discovered yet. Therefore, understanding of the role of geographical distribution and the prevalence pattern of stroke in different populations is important. The Stroke Belt in the US is not a geographically isolated island. It is an artificially grouped demographic area where stroke is more predominant than other states. Why stroke has prevailed in this geographical region was a mystery in 1951 and remains a mystery today. Similarly, more stroke cases are found in Alaskan natives compared to Caucasians in the US mainland [74]. Like Icelanders, they have had changes in lifestyle in recent decades with a shift to a Westernized diet. Though we have enough data on traditional risk factors in these regions, the lack of meta-data on new millennial factors in the stroke belt, stroke buckle and Alaska had crippled out further studies.
Predicting a forthcoming putative stroke insult in humans is difficult because of the multiple risk factors involved. Since 1970s, many molecules and genes were implicated for stroke and some were even statistically analyzed in the expectation of finding a diagnostic tool [75], but failed to come up with single solution. Until now, there is no indicative marker found for stroke. This is due to lack of its predictability in the context of the nature of stroke, as it is a polygenic disorder. Besides, stroke is a universal assailant without any discernment. It has prevailed globally, accounting for 5.7 million deaths worldwide and 16 million initial stroke attacks as reported in 2005. Since the traditional risk factors for stroke are similar in different parts of the world, [26], the pattern of distribution of the stroke should also be similar. However, as per INTERSTROKE study, all 22 countries including Asia (China, India, Pakistan, Philippines, Thailand, and Malaysia), Africa (Mozambique, Nigeria, South Africa, Sudan, and Uganda), Europe (Croatia, Denmark, Germany, Poland, Russia, Sweden, the UK, Ireland, and Turkey), the Middle East (Iran, Saudi Arabia, Kuwait, and United Arab Emirates), North America (Canada), Australia, and South America (Argentina, Brazil, Chile, Colombia, Ecuador, and Peru showed 47.9 % stroke outcome for just one risk factor, hypertension [26]. The GBD study considered 17 risk factors associated with stroke risk, based on systematic risk factors from earlier studies carried out in 188 countries [16]. However, it concluded that 67 % of stroke happens due to hypertension. In other words, variability pattern of prevalence of ischemic and hemorrhage strokes among population across countries and regions in all nations shows variability in causes, and not exclusive applicable to one risk factor. Although this study, by considering several genetic aspects of stroke, found that cardioembolic stroke is evidently consistent with PED4D associated, however, a robust genomic analysis of PDE4D should be commenced along with other parasol factors to detect genetic lesions of underlying disorder of stroke in the US and elsewhere worldwide.
Infection, Immune, Inflammation Factors
Stroke may occur due to underlying associations between physiological malfunctions of the immune system and infections of the brain [76]. Progression of latent infection depends on a number of factors of which the most important is the role of immune cells. An underlying immune-deficient state of an individual triggers a cascade of metabolic events followed by an infection that leads to inflammation and a higher prevalence of disorder. Interestingly commensal bacteria trigger exorbitant immune system activation inappropriately in those with chronic intestinal inflammation [77]. Individuals with these underlying immune malfunctions may end up with complicated metabolic modifications with a higher incidence of stroke development [78]. A bacterial infection causes immune cells to activate a multi-protein complex called the inflammasome [79]. Among other effects, it triggers the maturation of the enzyme Caspase-1, followed by the production of Interleukins such as IL-1β and IL-18 [80]. Macrophages and microglia are the major sources of IL1β within the ischemic brain [44]. Both Caspase-1 and IL-1β promote inflammation and cell death [81], thus, they directly associate with stroke insult in the brain [82, 83].
During a bacterial infection, the immune cells (the inherited T-cell and acquired B-cells) contribute to secondary neuro-degeneration [84] causing a variety of inflammatory stimuli [85] in brain tissues, which affects the ischemic areas [86] due to the release of neurotoxic factors such as reactive oxygen, nitrogen species, or exopeptidases, and locking the signals of inflammation has shown efficacy in stroke [87]. The regulation of cyclic AMP by PDE4D modulates inflammation and other processes, which affect atherosclerosis and stroke [88]. Therefore, inflammation is a key event in endothelial dysfunction [89]. It plays a role in the post-ischemic brain [90], showing subsequent brain damage [91] to promote stroke [92]. The present study confirms the association of infection, immune cells, and inflammation based on these meta-analyses.
Human Gut Microbiome Dysbiosis factor
The human gut is the most heavily colonized bacterial habitat in the entire body [93]. It is known that effectual dysbiosis (imbalance in ratio among bacteria) can trigger irritable bowel syndrome [94], Crohn's disease, and ulcerative colitis [95]. The gut microbiome modulates behaviors in neurodevelopmental disorders like autism and schizophrenia [96]. Recently, it is proved that dysbiosis of the gut microbiome with cerebral cavernous malformations (CCM) is involved in strokegenesis [78] by altering signaling pathways between gut microbes and the central nervous system via the vagus nerve [97, 98]. Humans harbor trillions of microbes, and some bacteria in the gut play a role in the formation of CCMs, a disease found among 1 in 100 people. CCMs are clusters of dilated, thin-walled blood vessels located in the brain that can cause stroke [78]. There is intensive up and down signaling between gut microbes and the brain via the central nervous system. CNS is composed of neural, immunological, and direct humoral signaling pathways [99]. The CNS creates dysbiosis by regulating gut motility and its mucosal immune response via the enteric nervous system and the neuronal-glial-epithelial unit [100, 101, 102]. In a reciprocate action, bacteria react to host hormones by sending neurotransmitters and/or neuromodulator signaling to the enteric nervous system (Figure 3) and stimulating signaling from the gut to the brain via the vagus nerves [103]. In the brain, the endothelial cells that underlie the formation of CCMs play a role in stroke. This is activated by TLR4 (Toll-Like Receptor-4). Lipopolysaccharides (LPS) can activate this TLR4 on brain endothelial cells, which forms the CCM. Midget bacteria, Gram negative bacteria in particular have LPS, which activates the TLR4, which may drive CCM formation. Thus, gut microbes play a key role in the pathology of CCM disease, which is a causative factor in certain kinds of stroke [78]. In addition, the PDE4 enzyme controls the level of cAMP and cGMP enzymes and they regulate many critical physiological functions [104] including but not limited to signal transduction, immune response, inflammation, neuronal activity, hypertension etc. They considered as the single most responsible metabolic factors in causing neurological disorders such as stroke in certain populations as revealed earlier in this study. Therefore inclusion of gut microbiome and PDE4D into SAM studies combined with other millennial risk factors is highly recommended as reliable predictive markers for cardioembolic stroke.
Conclusion
Current millennial research advancement has generated many genome-based revelations in causes and treatments of an array of diseases. It is moving rapidly into the era of medical-genome data analysis and personalized medicine due to the advancement in medical metadata and diagnostic biomarkers. In US, higher number of stroke events from newly emerging stroke regions such as Kentucky, West Virginia and Indiana are reported every day. The constant clinical monitoring of individuals, above 50 years of age, are recommended to ascertain for the sign and symptom of stroke annually before it victimize the individuals. We know it is difficult to prevent a stroke event, however it is possible to monitor high-risk individuals by taking appropriate preventive management by screening for Stroke Analysis Markers (SAM) during annual checkup or routine physical. In genetic counselling of an individual, SAMs could be used as a prognostic tool to help physicians to make an informed decision. Though significant challenges remain to be solved in early diagnostics, further large-scale studies on parasol factors will certainly unlock the secrets of early prediction of stroke. We believe this present study will potentially renovate the understanding of occurrence of stroke and will have an impact on promoting new biomarkers studies for better outcomes in stroke prevention.
Acknowledgements
The first author received a competitive research internship, under SIP of National Institutes of Health (NIH) and made substantial research on this topic at Bioinformatics and Computational Biosciences Branch (BCBB). The second author contributed this work while at NIH before move to the current position and he acknowledges the Dean of the college and Manipal University. The senior author acknowledges NIAID/NIH for a summer research support to work at BCBB-NIH. Dr. Darrell Hurt and Dr. Michael Dolan of Biomedical Informatics Program are gratefully acknowledged for their scientific supports including but not limited to lab space, computational equipment, critical analysis and constructive comments during and after research.
Competing Interests
The authors declare that the research was conducted in the absence of any commercial or financial relationships that could be construed as a potential conflict of interest. The content is solely the responsibility of the authors and does not necessarily represent the official views of the funding agencies. First author listed above made a very substantial, direct and intellectual contribution to the work, and all authors substantially contributed, mentored and approved it for publication.
References
1. WHO. The global burden of disease (2004 update). Geneva, Switzerland. http://www.who.int/healthinfo/global_burden_disease/GBD_report_2004update_full.pdf.
2. Zhao J, Mou Y. et al. Synthetic Oligodeoxynucleotides Containing Multiple Telemeric TTAGGG Motifs Suppress Inflammasome Activity in Macrophages Subjected to Oxygen and Glucose Deprivation and Reduce Ischemic Brain Injury in Stroke-Prone Spontaneously Hypertensive Rats. PLoSONE102006. 2015(10):e0140772. doi:10.1371
3. Mozzafarian D, Benjamin EJ, Go AS, Arnett DK, Blaha MJ, Cushman M. et al. On behalf of the American Heart Association Statistics Committee and Stroke Statistics Subcommittee. Heart disease and stroke statistics (2016 update): A report from the American Heart Association. Circulation. 2016;133(4):e38-360
4. Strong K, Mathers C, Bonita R. Preventing stroke: saving lives around the world. Lancet Neurol. 2007;6(2):182-187
5. Dawber TR, Meadors GF, Moore FE Jr. Epidemiological approaches to heart disease: the Framingham Study. Am J Public Health Nations Health. 1951;41(3):279-81
6. Xinxin Y, Xuelian T, Ping S, Yejie S, Kai L, Sulaiman HH. et al. MicroRNA-15a/16-1 Antagomir Ameliorates Ischemic Brain Injury in Experimental stroke. Stroke. 2017;48:1941-1947
7. Hall WD, Ferrario CM, Moore MA, Hall JE. et al. Hypertension-related morbidity and mortality in the southeastern United States. Am J Med Sci. 1997;313:195-209
8. Chambless LE, Heiss G, Shahar E, Earp MJ, Toole J. Prediction of ischemic stroke risk in the Atherosclerosis Risk in Communities Study. Am. J. Epidemiol. 2004;160:259-269
9. Cushman M, Cantrell RA, McClure LA, Howard G, Prineas RJ, Moy CS. et al. Estimated 10-year stroke risk by region and race in the United States: geographic and racial differences in stroke risk. Ann Neurol. 2008;64:507-513
10. Goyal M, Demchuk AM, Menon BK, Eesa M, Rempel JL, Thornton J. et al. Randomized assessment of rapid endovascular treatment of ischemic stroke. N Engl J Med. 2015;372:1019-1030
11. Bots ML, Elwood PC, Nikitin Y, Salonen JT, Freire de AC, Inzitari D. et al. Total and HDL cholesterol and risk of stroke. EUROSTROKE: a collaborative study among research centres in Europe. J Epidemiol. Community Health. 2002;56:19-24
12. Huang Y, Sharma VK, Robinson T, Lindley RI, Chen X, Kim JS. et al. Rationale, design, and progress of the enhanced control of hypertension and thrombolysis stroke study (ENCHANTED) trial: an international multicenter 2 × 2 quasi-factorial randomized controlled trial of low-vs.standard-dose rt-PA and early intensive vs. guideline-recommended blood pressure lowering in patients with acute ischaemic stroke eligible for thrombolysis treatment. Int J Stroke. 2015;10:778-788
13. NINDS (National Institute of Neurological Disorders and Stroke) rt-PA Stroke Study Group. Tissue plasminogen activator for acute ischemic stroke. N Eng J Med. 1995;333:1581-1587
14. Howard G. Why do we have a stroke belt in the southeastern United States? A review of unlikely and uninvestigated potential causes. Am. J. Med. Sci. 1999:317 160-167
15. Blauw GJ, Lagaay AM, Smelt AH, Westendorp RG. Stroke, statins, and cholesterol. A metaanalysis of randomized, placebo-controlled, double-blind trials with HMG-CoA reductase inhibitors. Stroke. 1997;28:946-950
16. Feigin VL, Roth GA, Naqhavi M. et al. Global burden of stroke and risk factors in 188 countries, during 1990-2013: a systematic analysis for the Global Burden of Disease Study Lancet Neurol 2013;16:30073-4.On line pub. http://dx.doi.org/10.1016/S1474-4422.
17. Chung JW, Bang OY Ahn K, Park SS Park T, Kim JG et al. Air pollution is associated with ischemic stroke via cardiogenic embolism. Stroke. 2016;48:STROKEAHA116. 015428
18. Pandian JD, Srikanth V, Read SJ, Thrift AG. Poverty and stroke in India: A time to act. Stroke. 2007;38:3063-9
19. WHO. Report on Task Force on Stroke and Cerebrovascular Diseases. Recommendations on stroke prevention, diagnosis and therapy. Stroke. 1989;20:1407-1431
20. D'Agostino RB, Wolf PA, Belanger AJ, Kannel WB. Stroke risk profile: Adjustment for antihypertensive medication. The Framingham study. Stroke. 1994;25:40-43
21. Manolio TA, Kronmal RA, Burke GL, O'Leary DH, Price TR. Short-term predictors of incident stroke in older adults. The Cardiovascular Health Study. Stroke. 1996;27:1479-86
22. George H, Roger A, Norman JJ, Paul S, Gregory R, Virginia J. Howard. Evaluation of Social Status as a Contributing Factor to the Stroke Belt Region of the United States. Stroke. 1997;28(5):936-940
23. Christopher TS, Diane EB, Teri AM. Overview of the Jackson Heart Study: A Study of Cardiovascular Diseases in African American Men and Women. Am J Med Sci. 1999;317(3):142-146
24. McClellan W, Warnock DG, McClure L, Campbell RC, Newsome BB, Howard V. et al. Racial differences in the prevalence of chronic kidney disease among participants in the Reasons for Geographic and Racial Differences in Stroke (REGARDS) Cohort Study. J Am Soc Nephrol. 2006:17 (6):1710-1715
25. Gaziano TA, Young CR, Fitzmaurice G, Atwood S, Gaziano JM. Laboratory-based versus nonlaboratory-based method for assessment of cardiovascular disease risk: The NHANES I follow-up study cohort. Lancet. 2008;371:923-931
26. O'Donnell MJ, Xavier D, Liu L. et al. Risk factors for ischaemic and intracerebral haemorrhagic stroke in 22 countries (the INTERSTROKE study): a case-control study. Lancet. 2010;376(9735):112-123
27. Hippisley CJ, Coupland C, Brindle P. Derivation and validation of Qstroke score for predicting risk of ischaemic stroke in primary care and comparison with other risk scores: a prospective open cohort study. BMJ. 2013;346:2573
28. Goff DC Jr, Lloyd-Jones DM, Bennett G, O'Donnell CJ, Coady S, Robinson J. et al. A 2013 ACC/AHA guideline on the assessment of cardiovascular risk: A report of the American College of Cardiology/American Heart Association task force on practice guidelines. J Am Coll Cardiol Circulation. 2014;129:S49-S73
29. Leslie AM, Dawn OK, Brett MK, Mary C, Elsayed ZS, George H. Assessing the Performance of the Framingham Stroke Risk Score in the Reasons for Geographic and Racial Differences in Stroke Cohort. Stroke. 2014;45:1716-1720
30. Anderson CS, Robinson T, Lindley RI, Arima H, Lavados PM, Lee TH. et al. Low-dose versus standard-dose intravenous alteplase in acute ischemic stroke. N Engl J Med. 2016;374:2313-2323
31. Sachdev P, Jessica WL, Crawford J, Mellon L, Hickey A, Williams D. et al. STROKOG (stroke and cognition consortium): An international consortium to examine the epidemiology, diagnosis, and treatment of neurocognitive disorders in relation to cerebrovascular disease. Alzheimer's & Dementia: Diagnosis, Assessment & Disease Monitoring. 2016 7: 10.1016/j.dadm.2016.10.006
32. Suwanwela NC, Poungvarin N. The Asian Stroke Advisory Panel. Stroke burden and stroke care system in Asia. Neurol India. 2016;64:Suppl S1:46-51
33. Pontes-Neto OM, Cougo P, Martins SC, Abud DG, Nogueira RG, Miranda M. et al. Brazilian guidelines for endovascular treatment of patients with acute ischemic stroke. Arq Neuropsiquiatr. 2017;75(1):50-56 (ISSN:1678-4227)
34. Dawber TR, Kannel WB, McNamara PM, Cohen ME. An epidemiologic study of apoplexy ("strokes"). Observations in 5,209 adults in The Framingham Study on Association of Various Factors in the Development of Apoplexy. Trans Am Neurol Assoc. 1965;90:237-240
35. Gretarsdottir S, Thorleifsson G, Reynisdottir ST, Manolescu A, Jonsdottir S, Jonsdottir T. et al. The gene encoding Phosphodiesterase4d confers risk of ischemic stroke. Nat. Genet. 2003;35:131-138
36. Jong SK, Yeon-Jung K, Kyung BL, Jae KC, Jong-Moo P, Yangha H. et al. Low- versus Standard-Dose Intravenous Alteplase in the Context of Bridging Therapy for Acute Ischemic Stroke: A Korean ENCHANTED Study. J of Stroke. 2018;20(1):131-139
37. Webportal #4. https://www.ncbi.nlm.nih.gov/pubmed/ PubMed comprises more than 27 million citations for biomedical literature from MEDLINE, life science journals, and online books. Citations may include links to full-text content from PubMed Central and publisher web sites.
38. Houslay MD, Adams DR. PDE4 cAMP Phosphodiesterase: modular enzymes that orchestrate signaling cross-talk, accession Numbers desensitization and compartmentalization. Biochem J. 2003:370 1-18
39. Graveley BR. Alternative splicing: increasing diversity in the proteomic world. Trends Genet. 2001;17:100-107
40. Gretarsdottir S. et al. Localization of a susceptibility gene for common forms of stroke to 5q12. Am J Hum Genet. 2002;70:593-603
41. Webportal #1. 1000 genome project browser. http://www.internationalgenome.org/home The International Genome Sample Resource (IGSR) helps to ensure the ongoing usability of data generated by the 1000 Genomes Project and to extend the data set.
42. Webportal #2 ensembl genome browser. http://www.ensembl.org/index.html Ensembl is a genome browser for vertebrate genomes that supports research in comparative genomics, evolution, sequence variation and transcriptional regulation.
43. Webportal #3. OMIM https://www.omim.org Online Mendelian Inheritance in Man (OMIM) is an Online Catalog of Human Genes and Genetic Disorders.
44. Lambertsen KL, Biber K, Finsen B. Inflammatory cytokinesin experimental and human stroke. J Cereb Blood Flow Metab. 2012;32:1677-98
45. Modrek B, Resch A, Grasso C, Lee C. Genome-wide detection of alternative splicing in expressed sequences of human genes. Nucleic Acids Res. 2001;29(13):2850-59
46. Emsley HC, Tyrrell PJ. Inflammation and infection in clinical stroke. J Cereb Blood Flow Metab. 2003;22:1399-1419
47. Boyd CD, Pierce RA, Schwarzbauer JE, Doege K, Sandell LJ. Alternate exon usage is a commonly used mechanism for increasing coding diversity within genes coding for extracellular matrix proteins. Matrix. 1993;13:457-469
48. Galante PAF, Sakabe NJ, Kirschbaum-Slager N, De Souza SJ. Detection and evaluation of intron retention events in the human transcriptome. RNA. 2004;10:757-765
49. Kate EK, Mary JK, Ted SA. Extracellular Matrix Gene Alternative Splicing by Trabecular Meshwork Cells in Response to Mechanical Stretching. IOVS. 2007;48(3):1164-1172
50. Klosin A, Casas E, Hidalgo-Carcedo C, Vavouri T, Lehner B. Transgenerational transmission of environmental information in C. elegans. Science. 2017;356:320-23
51. Lynch DC, Dyment DA, Huang L, Nikkel SM, Lacombe D, Campeau PM. et al. Identification of novel mutations confirms Pde4d as a major gene causing acrodysostosis. Hum Mutat. 2013;34:97-102 (Note: Erratum: 34:667)
52. Borhani NO. Changes and geographic distribution of mortality from cerebrovascular disease. Am J Public Health. 1965:55 673-681
53. Lanska DJ. Geographic distribution of stroke mortality in the United States: 1939-1941 to 1979-1981. Neurology. 1993:43 1839-1851
54. Perry HM, Roccella EJ. Conference Report on Stroke Mortality in the Southeastern United States. Hypertension. 1998;3:1206-15
55. Obisesan TO, Vargas CM, Gillum RF. Geographic variation in stroke risk in the United States. Region, urbanization, and hypertension in the Third National Health and Nutrition Examination Survey. Stroke. 2000;31:19-25
56. Smith SCJ, Zheng ZJ. The impending cardiovascular pandemic in China. Circ Cardiovasc Qual Outcomes. 2010;3(3):226-227
57. Mayowa OO, Oyedunni A, Sylvia M, Abiodun MA, Sally AA, Rufus A. et al. The burden of stroke in Africa: a glance at the present and a glimpse into the future. Cardiovasc J Afr. 2015;26(2):H3Africa Suppl): S27-S38. doi:10.5830/CVJA-2015-038
58. Shi1 JP, Chen WD, Zhou JQ, Xue MM, Xue F, Li HZ, Xu.ZP. Investigation of single nucleotide polymorphisms in phosphodiesterase 4D gene in Mongol and Han patients with ischemic stroke in Inner Mongolia. Genetics and Mol Res. 2015;14(3):10281-10287
59. Elin L, Andreas Gs, Jakob CM, Tõnis O, Erich W, Gerhard H, Thomas M, Martin D. ALOX5AP Gene and the PDE4D Gene in a Central European Population of Stroke Patients. Stroke. 2005;36:731-736
60. Myeong-Kyu K, Jun-Tae K, Sung-Min C, Seung-Han L, Man-Seok P, Ki-Hyun C. Phosphodiesterase 4D Gene and Risk of Noncardiogenic Ischemic Stroke in a Korean Population. J Korean Med Sci. 2009;24:307-10
61. Corbin JD, Francis SH. Cyclic GMP phosphodiesterase-5: target of sildenafil. J Biol Chem. 1999;274:13729-32
62. Kam YZ, Graeme LC, Suzuki Y, Richard A, Daniel F, Sam G. et al. A Glutamine Switch Mechanism for Nucleotide Selectivity by Phosphodiesterases. Mol Cell. 2004;15:279-286
63. Shao MJ, Yi XY. ALOX5AP and PDE4D gene polymorphisms and ischemic stroke. Int J Cerebrovasc Dis. 2012;20:621-626
64. Gudbjartsson DF, Patrick S, Hannes H, Arnaldur G, Sigurjon AG, Florian Z. et al. Sequence variants from whole genome sequencing a large group of Icelanders. Scientific Data. 2015:2 :150011; DOI: 10.1038/sdata.2015.11 (www.nature.com/scitificdata)
65. Kriventseva EV, Koch I, Apweiler R, Vingron M, Bork P, Gelfand MS, Sunyaev S. Increase of functional diversity by alternative splicing. Trends Genet. 2003;19:124-128
66. Brenton R. Graveley. Alternative splicing: increasing diversity in the proteomic world. Trends in Genetics. 2001;17(2):100-107
67. Gilbert W. Why genes in pieces? Nature. 1978;271(5645):501
68. Roland WB, Elizabet T, Vahri B. Role of Histone Deacetylases in Neurodegenerative diseases and small molecule inhibitors as potential therapeutic approach. Top Med Chem. 2010;6:1-56
69. Campbell ID. Modular proteins at the cell surface. Biochem. Soc. Trans. 2003;31:1107-14
70. Mecham RP. The extracellular matrix: an overview. 2011;(Ed.) New York: Springer.
71. Hohenester E. and Engel, J. Domain structure and organization in extracellular matrix proteins. Matrix Biol. 2002;21:115-128
72. Patthy L. Modular assembly of genes and the evolution of new functions. Genetica. 2003;118:217-231
73. Ast G. How did alternative splicing evolve? Nat Rev Genet. 2004;5:773-782
74. Truelsen T. Advances in population-based studies. Stroke. 2010;41(2):e99-101
75. Matarín M, Mark BW, Sonja S, Simón-Sánchez J, Hon-Chung F, Dena H. A genome-wide genotyping study in patients with ischaemic stroke: initial analysis and data release. Lancet Neurol. 2007;6:414-20
76. Sartor RB. Microbial factor in the pathogenesis of Crohn's disease, ulcerative colitis, and experimental intestinal inflammation in: Kirsner JB (Ed.) Inflammatory Bowel Disease. 2000. WB Saunders, Philadelphia:153-178.
77. Farrell R.J LaMont JT. Microbial factors in inflammatory bowel disease. Gastroenterol. Clin. North Am. 2002;31:41-62
78. Alan TT, Jaesung PC, Jonathan JK, Yiqing Y, Courtney CH, Nicholas H, et al.Endothelial TLR4, the microbiome drive cerebral cavernous malformations. Nature 2017;545:305-310.
79. Schroder K, Tschopp J. The inflammasomes. Cell. 2010;140:821-832
80. Latz E, Xiao TS, Stutz A. Activation and regulation of the inflammasomes. Nat Rev Immunol. 2013;13:397-411
81. Dinarello CA. A clinical perspective of IL1 beta as the gate keeper of inflammation. Eur.J. Immunol. 2011;41:1203-1217
82. Bergsbaken T, Fink SL, Cookson BT. Pyroptosis: host cell death and inflammation. Nat.Rev. Microbiol. 2009;7:99-109
83. Denes A, Lopez-Castejon G, Brough D. Caspase-1 is IL1just the tip of the Iceberg? Cell Death Dis. 2012;3:e338.doi 10.1038/cddis.2012.86PMID:22764097
84. Katarzyna W, Andreas M, Ulrich D. Gut microbiota impact on stroke outcome: Fad or fact? J Cerebral Blood Flow & Metabolism. 2016;36(5):891-898
85. Martin V, John DD, Megan MR, Irene G, Rachael F, Ben AB, Joshua PT. Microglial Inflammatory Signaling Orchestrates the Hypothalamic Immune Response to Dietary Excess and Mediates Obesity. Susceptibility. 2017;26(1):185-197
86. Yang T, Huichun X, Xin-Li Du, Lisa L, Wynn W, Aigang L. et al. Gene expression in blood changes rapidly in neutrophils and monocytes after ischemic stroke in humans: a microarray study. J Cerebral Blood Flow & Metaboli. 2006;26:1089-1102
87. Iadecola C, Anrather J. The immunology of stroke: from mechanisms to translation. Nat Med. 2011;17:796-808
88. Eric JH, Joanna LM, Justin TW, Hani RM, Christopher K, Samuel SB, Sander EC. Phosphodiesterase 4D Single-Nucleotide Polymorphism 83 and Cognitive Dysfunction in Carotid Endarterectomy Patients. Neurosurgery. 2013;73(5):791-796
89. Robert GW, Laura CT, Marc-Antoine C, Niall M, Philip MC, Marco Mangone, Ronan PM. Potential Diagnostic and Prognostic Biomarkers of Epigenetic Drift within the Cardiovascular Compartment. Biomed Res Int. 2016;246:5763
90. Henninger N, Kumar R, Fisher M. Acute ischemic stroke therapy. Expert Rev Cardiovasc Ther. 2010;8:1389-98
91. Fugate JE, Lyons JL, Thakur KT, Smith BR, Hedley-Whyte ET, et.al. Infectious causes of stroke. Lancet Infect Dis. 2014;14:869-880
92. Courties G, Moskowitz MA, Nahrendorf M. The innate immune system after ischemic injury: lessons to be learned from the heart and brain. JAMA Neurol. 2014;71:233-236
93. Derrien M, Vlieg van HJE. Fate, activity, and impact of ingested bacteria within the human gut microbiota. Trends Microbiol. 2015;23:354-366
94. Rajilic-Stojanovic M, Biagi E, Heilig HG, Kajander K, Kekkonen RA, Tims, S. Global and deep molecular analysis of microbiota signatures in fecal samples from patients with irritable bowel syndrome. Gastroenterology. 2011;141:1792-1801
95. Podolsky DK. Inflammatory bowel disease. N Engl J Med. 2002;347:417-429
96. Mayer EA, Knight R, Mazmanian SK, Cryan JF. Gut microbes and the brain: paradigm shift in neuroscience. J Neurosci. 2014;34:15490-15496
97. Joutel A, Corpechot C, Ducros A. et al. Notch3 mutations in CADASIL, a hereditary adult-onset condition causing stroke and dementia. Nature. 1996;383:707-10
98. Helgadottir A, Andrei M, Gudmar T, Solveig G, Helga J. et al. The Gene Encoding 5-Lipoxygenase Activating Protein Confers Risk Of Myocardial Infarction And Stroke. Nature Genetics. 2004:36 (3); 233-239
99. Collins SM, Surette M, Bercik P. The interplay between the intestinal microbiota and the brain. Nat Rev Microbiol. 2012;10:735-742
100. Furness JB. The enteric nervous system and neurogastroenterology. Nat Rev Gastroenterol Hepatol. 2012;9:286-294
101. Matteoli G, Boeckxstaens GE. The vagal innervation of the gut and immune homeostasis. Gut. 2013;62:1214-22
102. Neunlist M, Landeghem VL, Mahe MM. The digestive neuronal-glial-epithelial unit: A new actor in gut health and disease. Nat Rev Gastroenterol Hepatol. 2013;10:90-100
103. Forsythe P, Kunze WA. Voices from within: Gut microbes and the CNS. Cell Mol Life Sci. 2013;70:55-69
104. Conti M, Richter W, Mehats C. et al. Cyclic AMP-specific PDE4 phosphodiesterases as critical components of cyclic AMP signaling. J Biol Chem. 2003;278:5493-5496
Author contact
Corresponding author: Prof. Narayanan Rajendran PhD, Professor, Molecular Microbiology, School of Natural Sciences, Kentucky State University, Frankfort, KY 40601. narayanan.rajendranedu